AI's Quantum Leap for the Curator Economy
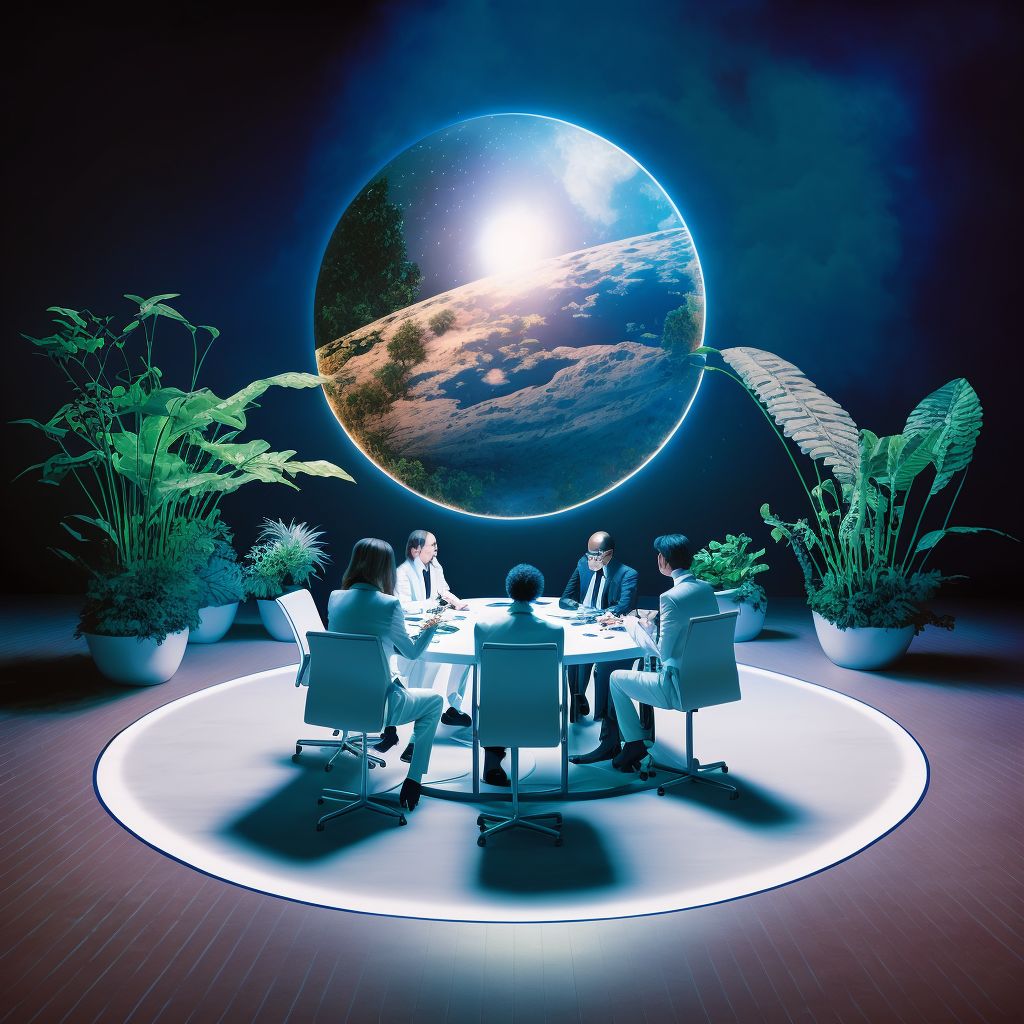
The sunlight glimmers off the table as I tentatively offer more than ask my first question. I look across my table of dissenting opiners, each trained in a different and important tradition of human knowledge. The infinitely well-researched and well-reasoned experts span philosophy, ethics, science, medicine, business, design, mysticism, architecture, botany, music, technology, ecology, history, literature, psychology, law, mathematics, art, and policy. They never tire of my boundless curiosity, and I never tire of their wealth of wisdom.
Every follow-up question I ask is considered from each relevant vantage point with perfectly crafted reasoning and source documentation. I prompt my logician and statistician to synthesize the answers complete with rank-ordered probabilities of success. After a brief moment of deep reflection, I land upon my ultimate decision - yes, golden eye eggs for breakfast today.
Then I'll tackle my delusions of grandeur by solving Thriving for humanity's first interplanetary epoch.
With my panels of carefully curated and trained AI confidant-bots, I can unlock new worlds previously inaccessible.
—
November 30th, 2022 - the day of the keystroke felt around the world. OpenAI, the creator of ChatGPT, publicly released its first chatbot powered by its GPT 3 model and ramped to 1 million users within 5 days. Within 2 months, it was 100 million users. Some predict by the end of 2023 it'll be 1 billion users.
In a recent interview with Cade Metz of the New York Times, Sam Altman, the CEO of OpenAI, suggested that artificial general intelligence (AGI) will radically redistribute wealth in the global economy. His guess was somewhere between $1 trillion to $100 trillion dollars. That is ~1% to *ahem* 100% of global GDP in 2022.
Like many, I have extremely mixed feelings about current capabilities, let alone where we are headed. One area I find especially troubling is the proliferation of mediocre regurgitation (at best) or active misinformation (at worst). That means signal-to-noise will plummet and careful curation will become ever more important.
Not surprisingly, Generative AI is an integral part of the problem and the solution.
Content overload leads to cognitive overload
We live in an era of content gluttony. The widespread adoption of social media significantly lowered the barrier to entry for individuals to create and post content. Each platform's feed algorithm is a hungry ghost that can never be sated. And neither can we.
The upside is that the scope of human knowledge is both greater and more accessible than at any time in history. The downside is that parsing all those bits to find something relevant AND trustworthy gets harder every day.
Some fun stats - every minute of every day ...
- 500 *hours* of video is uploaded to YouTube
- 175 new websites are created
- ~4 peer-reviewed articles are published
- ~1700 news articles are published
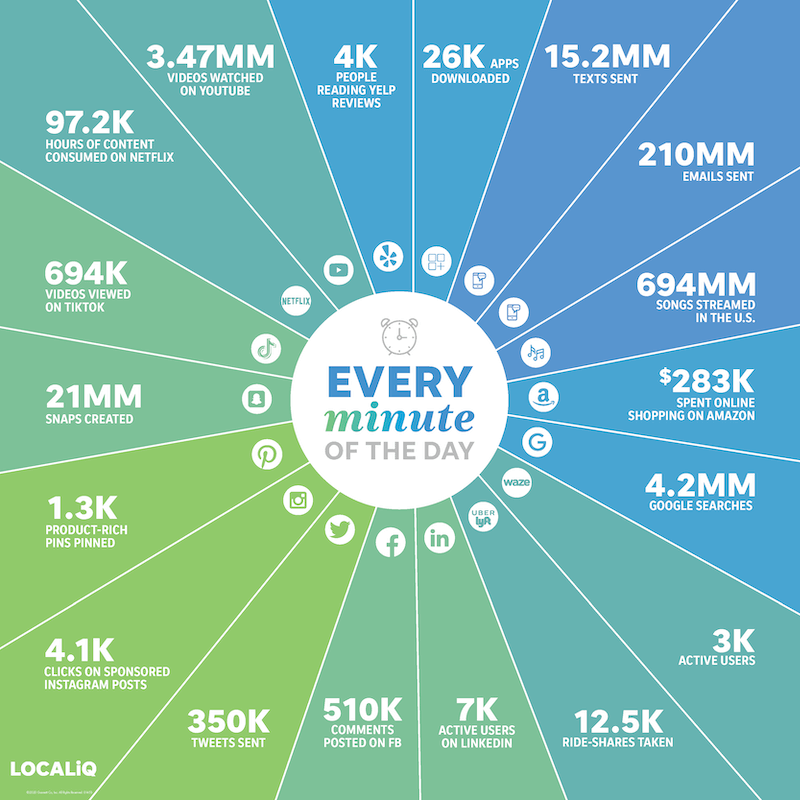
My overloaded point is that we all create radical exclusion filters to survive this deluge of content. A select few pick fixed publications or information streams and don't stray afield. The vast majority abdicate their information filters partially or entirely to algorithms.
When it's puppy videos or pretty people selling me things I don't need but should definitely buy, no big deal. But when it comes to factual or meaningful information, trust becomes paramount.
Trust is a scarce commodity online, and it's getting scarcer
Fake news is real. Before social media, misinformation campaigns were often relegated to clandestine intelligence agencies and propaganda machinery. Today, as evidenced by Donald Trump throughout his election and beyond, a steady hose of factually inaccurate or misleading tweets can dilute or pollute real information at a massive scale for a fraction of the cost and complexity. This muddying of the digital stream creates confusion, uncertainty, anger, and distrust.
About 60% of Americans get their news from social media. With skillful manipulation, expert data nerds can take our digital exhaust, create psychographic profiles, and systematically manipulate our thoughts and beliefs (thanks Cambridge Analytica!). On top of that, fewer than 1 in 4 Americans avoid echo chambers and verify information.

Then came the COVID-19 pandemic that further eroded trust in public institutions. And the general trend of dropping trust becomes precipitous in conservative and certain ethnic communities.
So the majority distrust institutional, social, and search sources then amplify their "trusted" sources without questioning or fact-checking. Trusting anything on social media or published online is default suspect without the onerous task of rigorous fact-checking and sensemaking.
In the good old days of baby blood-drinking cabal conspiracies, misinformation was mostly obvious and easy to spot. Generative AI is complicating the matter tremendously.
GPT sounds legit, but it's trippin' (right?)
Generative AI, and specifically ChatGPT, has become a new source and creator for millions of global citizens. It answers questions with stunning speed and certainty. And the answers generally sound right to all but the most trained expert.
These "sounds right, right?" wrong answers are technically called hallucinations, and there is currently no way to gauge their frequency.
ChatGPT misinformation is particularly alarming for a number of reasons:
- It gets many things right, so a halo effect leads to misplaced trust in subsequent queries
- The tone can come across as very confident even when it's profoundly wrong, and confidence breeds trust
- Most people never ask for sources, and for those that do ChatGPT can hallucinate very real-sounding citations
- Even those that want to fact-check may struggle when answers are a synthesis of disparate sources and ideas
And now that the internet is becoming flush with mediocre and vaguely (in)accurate AI-generated content, the AI hall of mirrors can cite articles and posts that are themselves poorly researched hallucinations. One or two legit-sounding articles linking to other legit-sounding articles that all boil down to an uncited (hallucinated?) source and voila! There is effectively no way to grasp what is real online anymore.
Beyond appropriately trusted sources, of course.
Case in point: Back in Aug 2022, an author cheerfully celebrated his vapid Curator Economy article as being 99% created by AI. He / it references "the new economy," without a link or citation, as "we're all connected and everything is hyper-connected". In fact, the economist Edward Deming's seminal book on the topic has nothing to do with all connected hyper-connectedness. I hope to all that is good and just that Bing, Bard, and web-plugged GPT can exclude or down-regulate garbage fluff in their training sets.
Curation isn't new - it's just experiencing a quantum leap with GPT
Curation has been around as long as humans have sought power through information asymmetry. Carefully curated libraries were a signal of wealth and prestige. As technology progressed toward more affordable papers and books, editors and publishers curated the content fed to the masses. Certain publications and publishing houses proactively made choices to filter content deemed not up to rigorous research and intellectual integrity standards. Peer-reviewed academic journals, at least in theory, are curated for well-crafted experiments that meaningfully add to human knowledge. Most curation processes introduce biases and decrease the scope and rate of published content. The most rigorous and prestigious curators have a robust selection process that ensures quality and trustworthiness.
Nowadays, we have a cottage industry of email newsletters (much like my own Thriving Thursday) and hundreds of thousands of aggregator channels on every social platform. Each curator picks a relatively narrow scope to start and catch the attention of a small audience. As the audience grows, the curator tries to get more interesting to more people at the margin and tends to grow and expand the things they cover. If they get big enough, they can start selling ads or subscriptions to make money.
Curation is incredibly time-consuming, partly because it requires a human to read / watch / listen to the source material and decide if it's relevant and interesting to their audience. Oftentimes, the curation comes with some sort of summary or synthesis to create a roadmap to relevant, interesting content. That summary or synthesis is sometimes valuable and sometimes entertaining but always seen through the lens of the curator.
GPT turns this whole equation on its head. The role of the curator is rapidly evolving. Looking ahead,
- Curators will operate at a much larger scale with greater breadth and depth of materials
- Curators will use Gen AI to summarize and cross-check new information against existing trusted streams
- Curators will spend much less time reading source material and writing and much more time validating and fact-checking based on generated summaries
- Readers / listeners will ask questions directly in the voice and framing that is most resonant to them
- Curators will take the revealed preferences from the plethora of log data to source and feed additional relevant, trustworthy information
Above all else, curators will transact in the currency of trust. The four pillars of curator trust are:
- Transparency - Publish selection criteria for what makes the "trust cut" as well as the full list of trained materials in the catalog
- Verifiability - Ensure the AI only primarily (entirely?) relies on the training corpus, cites all its sources, and all the cited sources are real
- Consistency - Continually staying current and responsive to evolving needs of the audience base
- Confidence - Explicitly assign some confidence interval based on the rigor and quantity of relevant source materials
The skills needed to be a curator of tomorrow are vastly different than those sending out newsletters with 3-5 links today. And those who master these pillars will reap the rewards within Altman's prophesied redistribution of wealth.
A few early-stage examples
Dan Shipper of Every has been the most influential writer I've read making the point of AI-powered curation and synthesis. Starting with personal knowledge management and expanding to other use cases, Dan has argued that AI sidekicks will consume our personal data and our personal trusted streams to make them more accessible and useful.
His first few prototypes were HubermanBot and LennyBot. Granted, when I ask relatively basic synthesis questions I get a "I'm sorry, I can't help with that" answer, but it's definitely a step in the right direction.
Dan's early work inspired me to build PIE Bot - a rough alpha that repeatedly trips up. It even hallucinated a citation to a legit-looking journal article with a DOI number and a journal link that doesn't exist! But it gets most things right most of the time, and that's a step in the right direction.
These are all toy applications to prove a point - parlor tricks that foreshadow fascinating applications. Yohei Nakajima, a pre-seed stage VC, is already using GPT to automate and systematize vast troves of knowledge-based work for his fund. The multiple models and feedback loops he's created are a brilliant way to train his AI to maximize value for his founders and his funders.
Wait, what about the creators?
This opens a whole new branch of ethical and economic considerations around monetizing the curation of content as distinct from the creation of content.
Every author has read extensively in their life. Their creative output is an inexplicable amalgamation of their creative inspirations plus their own unique contributions. When I read then mimic my favorite authors, I practice my craft. As long as I cite quotes and don't lift passages wholesale, I'm well within copyright bounds to synthesize and create something novel. The same thing is true when I publish book notes and companies like CliffsNotes made a lucrative business summarizing texts for lazy students (🙋).
Does it work the same way if I train a large language model (LLM) to "read" a bunch of creators (authors, podcasters, etc) then "write" or summarize something novel? How about if it’s in the format of a conversational chat?
If Dan wanted to monetize his HubermanBot, does that violate copyright? And even if laggard laws can't answer that, is it unethical? Should Huberman get a cut of the proceeds since it's his content doing the answering? And if so, how much is appropriate compensation for the source content? Clearly, there is value in building the AI wrapper, but how should creator and curator share the profit stream?
And how will all this play out in copyright court? Is an AI building K neighborhoods based on semantic lat-longs equivalent to undue plagiarism? If this is similar to how humans learn and write, is the problem the vast speed and scale of digesting information? And since LLMs are fields of statistical probability drawing upon millions or billions of data points to join words often written together, what does copyright infringement even mean?
Back to the future
In Dan Simmons' 4-part Hyperion Cantos, humans create an AI that becomes the eyes, ears, and hands of humanity across the universe. I often think about the scenes where various AI entities do exceptionally complex computations and logical constructs in a conversational tone with human collaborators.
For every major or minor decision I need to make or idea I want to explore, I can draft a list of domain experts I want on my team. To qualify them as subject matter experts, I can draft a corpus of curated knowledge across time, platform, discipline, and media. Based on rigorous rationality and source metadata, I can create confidence intervals to weigh logical conclusions. Each response or answer can come complete with how likely it is to be true or accurate given the best awareness in the training set. Based on my worldview and belief system, I may weigh different ideas or domains differently in balancing competing interests to construct my final answer. Throughout the process, I can ask my AI experts to suggest new elements to add or consider.
As I gain confidence in the transparency and consistency of answers, I can open my tools to the public to start expanding the set of questions and use cases for various trained "experts". Similar to Yohei’s feedback loops, it’s possible to create a system where the collective conscious of questions feeds every greater clarity of what we truly understand and what needs to be studied further.
Assuming the curation can be done ethically and responsibly, these well-researched and always-citing AI councilors have the potential to systematically solve the first Thrive Blocker. The other two blockers will likely still need some (AI-enabled) human support for emotional and social reasons.
Fast forward to now
This potential application is crystal clear, but the technology isn't there today. For one thing, I can't get GPT to reliably only answer from the corpus I've trained it on. For another, I can't get it to cite sources that are real and within my corpus. Practically, that means most of my queries in GPT are simply a starting point for further research (that regularly leads to blunt falsehoods and dead ends).
It's possible that ChatGPT's open-source retrieval plugin may solve some of these concerns. Then again, at least one expert pointed out the frequent hallucinations in the plugins demo video.
OpenAI's indelible mark has created a Cambrian explosion of for-profit, public benefit, and non-profit entities spinning up their own counterpoint LLM offerings. I am generally optimistic market forces will nudge a provider to create a solution aligned with my proposed panel of "Curated AI Experts" use case for business purposes (similar to BloombergGPT). It's just a matter of time, and I'll keep fiddling with the tech as best I can to create the B2C and B2B Thriving-focused versions of my vision.
Now if only I could trust GPT's recommendation for my lunch ...